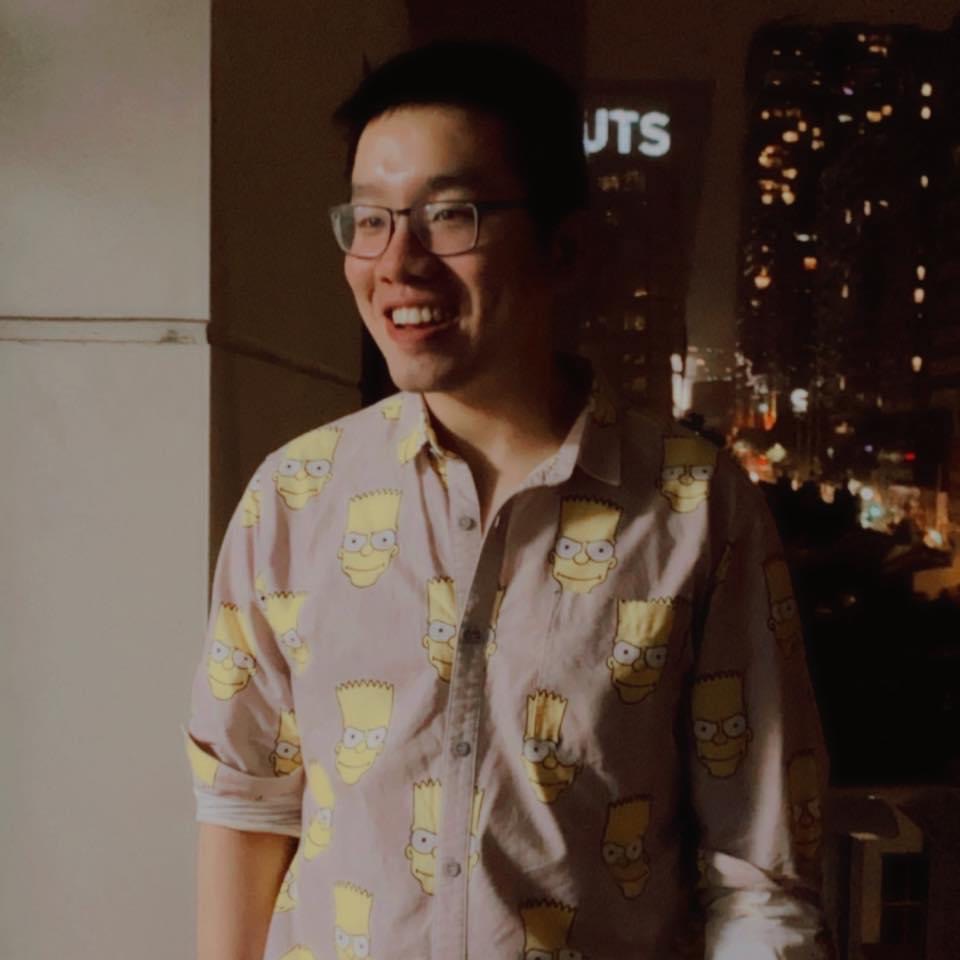
Zhuowei Wang (Commonwealth Scientific and Industrial Research Organization, Australia)
Zhuowei Wang is a postdoctoral fellow at Commonwealth Scientific and Industrial Research Organization (CSIRO), Australia. Prior to joining CSIRO, he was with the Australian Artificial Intelligence Institute (AAII), University of Technology Sydney. His current research interests include weakly-supervised learning, noisy label learning, and federated learning. Recently, he will be transferring his research to collaborate intelligence applications.
Short Abstract: The success of deep learning depends on the correct annotation of all training samples for training robust models, where samples are difficult and expensive to obtain. The incorrect or incomplete information brought by wrongly annotated labels may cause catastrophic effects depending on the real-world applications. Therefore, this talk introduces a framework using semi-supervised learning to tackle two different kinds of weakly supervised learning, noisy label learning (NLL) and positive unlabeled learning (PUL), to improve the model robustness under incorrect labels in the dataset. Moreover, in real-world scenarios, most data are not collected and stored in a centralized way. Instead, data are distributed over various institutions protected by privacy restrictions. Federated learning (FL) has been proposed to leverage isolated data without violating privacy. However, data labels in different institutions are not annotated according to the same criterion so they inevitably contain different noises across silos. The frame can also be applied to tackle NLL problem in the FL setting.