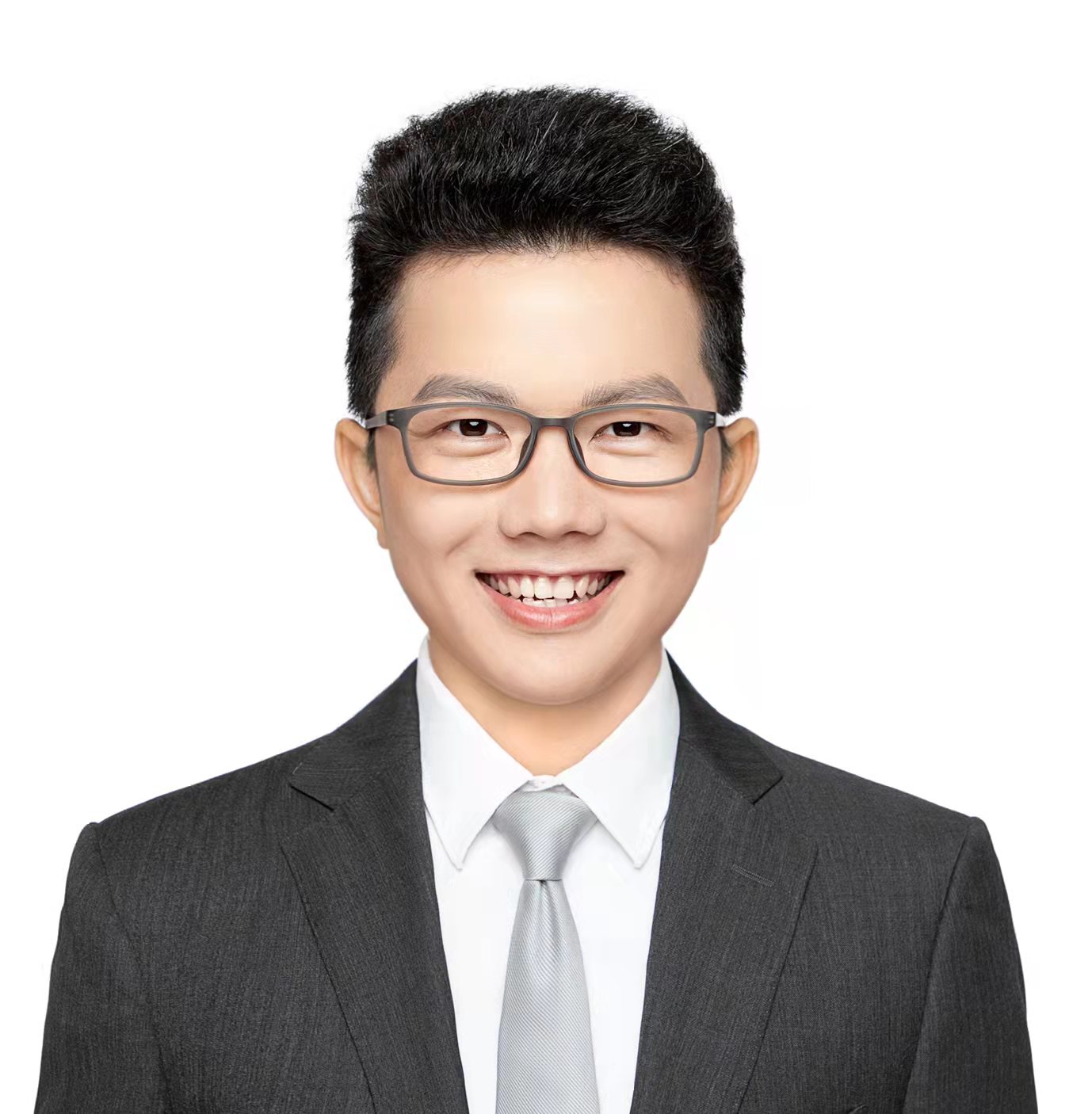
Ximei Wang (Tsinghua University)
I am currently a Ph.D. candidate in the School of Software, Tsinghua University, advised by Prof. Jianmin Wang and Prof. Mingsheng Long. Before that, I received my B.S. degree from the Department of Automation, Tsinghua University. My research interests lie in transfer learning, domain adaptation, and semi-supervised learning. I am an outstanding reviewer for NeurIPS 2020, ICLR 2021, and NeurIPS 2021, and also an expert reviewer for ICML 2021.
Short Abstract: The fundamental assumption in traditional machine learning that training and test data are drawn from identical distribution may be too restricted to be satisfied in real-world applications. To this end, transfer learning is proposed to reduce the dataset shift across distributions and transfer knowledge from a related domain/model to a target task. In this talk, I will first give a brief introduction to the two main paradigms of transfer learning: domain adaptation and task adaptation. Then, I will delve into the challenges and advances of them from the perspectives of transferable normalization and self-tuning respectively. Finally, I will summarize some possible research directions in this field.