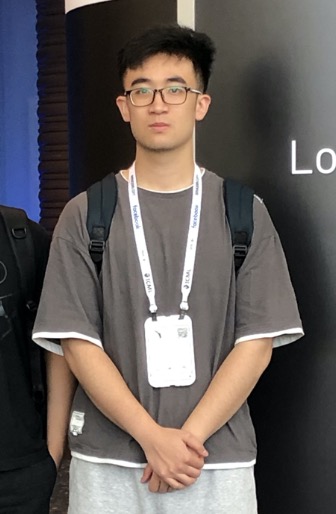
Tianyu Pang (Tsinghua University & Sea AI Lab)
Tianyu Pang is a final-year PhD student in the Computer Science Department at Tsinghua University, advised by Prof. Jun Zhu. He received B.S. degree of Mathematics and Physics from Tsinghua University in 2017. His research interests include machine learning and computer vision, especially on the trustworthy aspects. His research is supported by Microsoft Research Asia Fellowship and Baidu Scholarship.
Short Abstract: In the adversarial literature, the prevailing view believes that the trade-off between robustness and accuracy is inherent. In this talk, we challenge this view from both practical and theoretical aspects. First, we show that robust learning is sensitive to implementation details, and we provide comprehensive studies on a bag of empirical tricks. Second, we find that the robustness-accuracy trade-off may stem from the improper definition of robust error, while a properly self-consistent robust error (SCORE) principally reconciles robustness with accuracy. SCORE also provides interesting insights, towards explaining overfitting and semantic gradients encountered in robust learning.