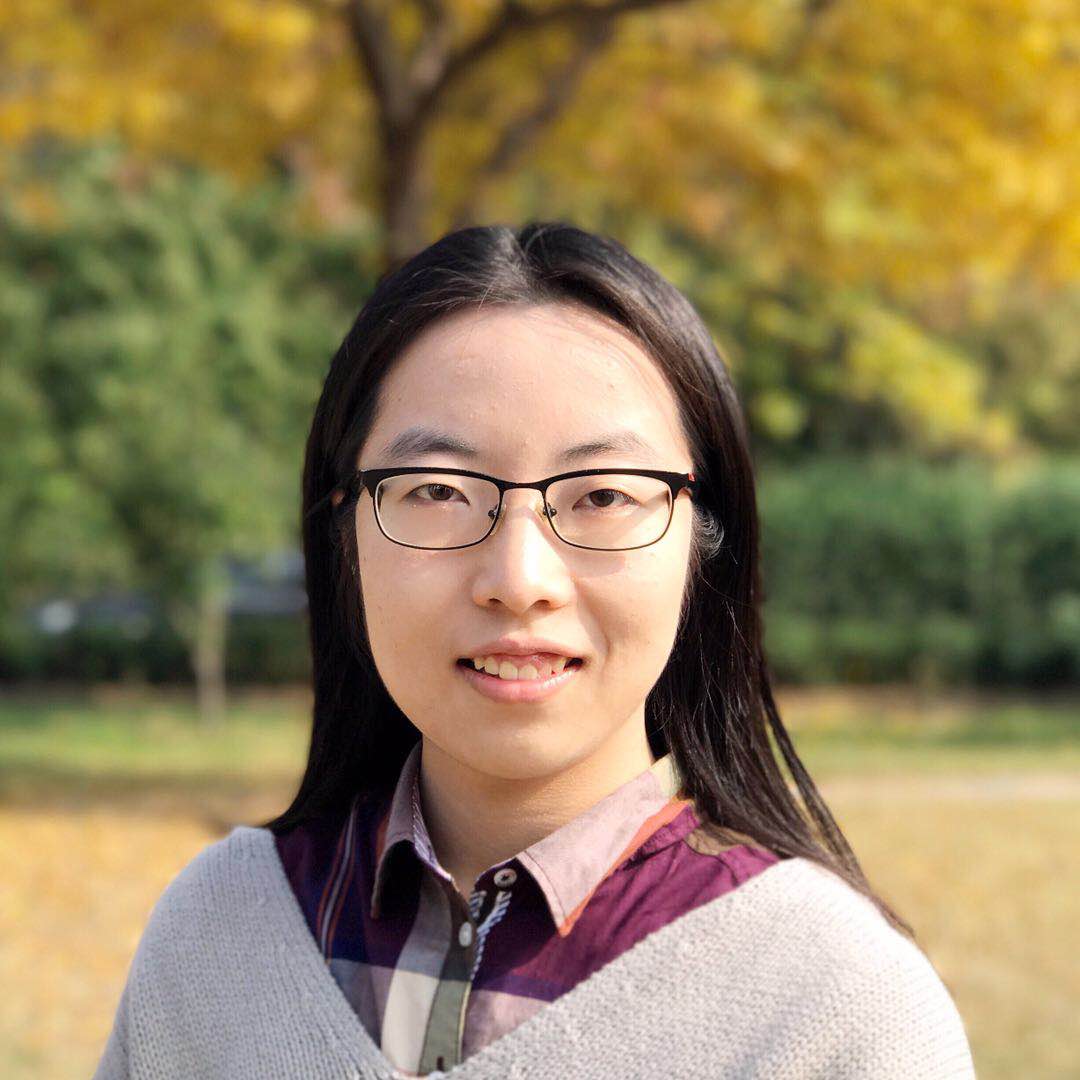
Tian Li (Carnegie Mellon University)
Tian Li is a Ph.D. student in the Computer Science Department at Carnegie Mellon University working with Virginia Smith. Her research interests are in distributed optimization, federated learning, and data-intensive systems. Prior to CMU, she received her undergraduate degrees in Computer Science and Economics from Peking University.
Short Abstract: Fairness and robustness are two important concerns for federated learning systems. We identify that robustness to data and model poisoning attacks and fairness, measured as the uniformity of performance across devices, are competing constraints in statistically heterogeneous networks. In this talk, I talk about a simple, general framework for personalized federated learning, Ditto, that can inherently provide fairness and robustness benefits, and a scalable solver for it. I then talk about the theoretical analyses to showcase the ability of Ditto to achieve fairness and robustness simultaneously on a class of linear problems. Finally, I talk about empirical results---across a suite of federated datasets, Ditto not only achieves competitive performance relative to recent personalization methods, but also enables more accurate, robust, and fair models relative to state-of-the-art fair or robust baselines. I conclude the talk with future works.