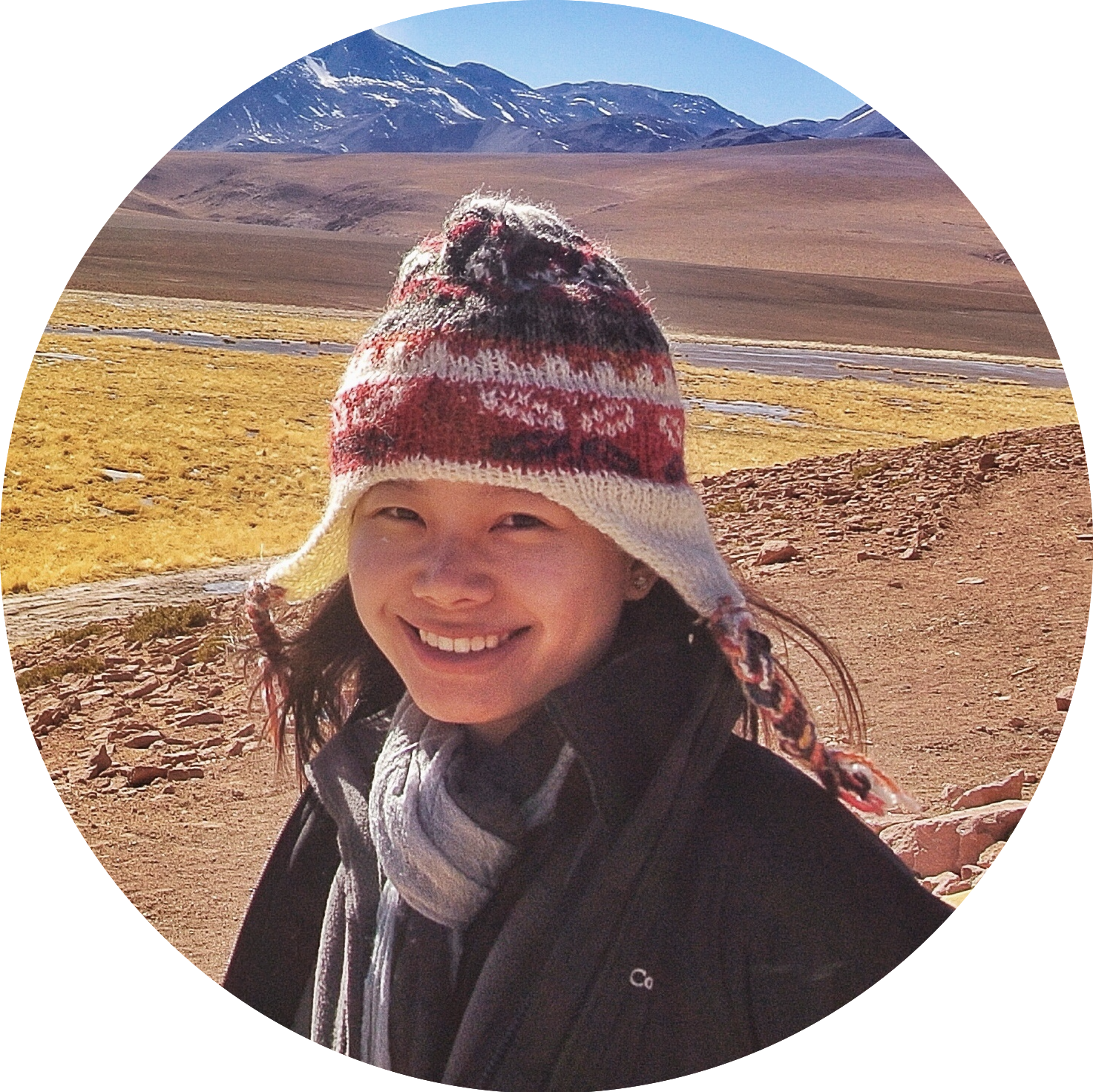
Teresa Yeo (EPFL)
Teresa Yeo is a Ph.D. student at École polytechnique fédérale de Lausanne supervised by Amir Zamir and Pierre Dillenbourg. She received her bachelor's and master's degree from the National University of Singapore and University of Cambridge. Her interest is in building robust and adaptive computer vision models.
Short Abstract: Neural networks are able to learn complex functions on in-distribution data. However, their predictions are unreliable under distribution shifts, i.e. they are not robust. In this talk, I will present a method for making neural network predictions robust to shifts from the training data distribution. The proposed method is based on making predictions via a diverse set of cues and ensembling them into one strong prediction. The merging is done in a straightforward but principled manner based on the uncertainty associated with each prediction. Evaluations performed on multiple tasks and datasets under a wide range of shifts demonstrate that the proposed method is more robust than the baselines.