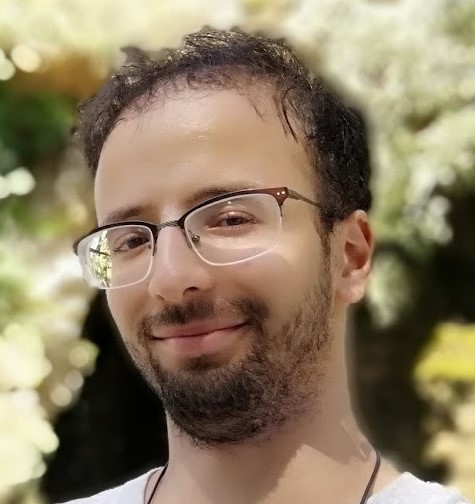
Salah Ghamizi (University of Luxembourg)
Salah Ghamizi has recently completed his PhD thesis at the University of Luxembourg and is currently a post-doctoral researcher at Interdisciplinary Center for Security, Reliability and Trust (SnT). His research focuses practical solutions to achieve robust machine learning, using self-supervised learning and multi-task learning. His work lie at the intersection of Software Security and Machine Learning, with his recent publications presented in venues such as ICCV, IJCAI, AAAI, KDD (Best paper award), and S&P.
Short Abstract: With the heavy reliance on Information Technologies in every aspect of our daily lives, Machine Learning (ML) models have become a cornerstone of these technologies’ rapid growth and pervasiveness. In particular, the most critical and fundamental technologies that handle our economic systems, transportation, health, and even privacy. However, while these systems are becoming more effective, their complexity inherently decreases our ability to understand, test, and assess the dependability and trustworthiness of these systems. This problem becomes even more challenging under a multi-objective framework: When the ML model is required to learn multiple tasks together, behave under constrained inputs or fulfill contradicting concomitant objectives. In this talk, we introduce the concept of "Knowledge Augmentation", a novel set of approaches to improve the robustness and performances of ML in the Real World. The talk will cover three real use cases: Fraud detection, pandemic forecasting, and medical diagnosis.