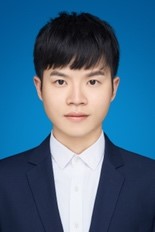
Mouxing Yang (Sichuan Univerisity)
Mouxing Yang is currently pursuing the Ph.D. degree at the College of Computer Science, Sichuan University. His work focuses on multi-modal learning and label noise learning, especially the combination of both.
Short Abstract: In this talk, I will present a new paradigm for label noise, namely, Learning with Noisy Correspondence. Specifically, the widely-studied label noise usually focuses on the category-level annotation errors and most existing studies take classification as the evaluation scenario. In contrast, noisy correspondence refers to the matching errors in paired data such as the mismatch pairs in cross-modal retrieval and wrongly-related negatives in contrastive learning. For a systematic study, I will introduce three recent works which study noisy correspondence from the aspects of false negatives in contrastive learning, false positives in cross-modal retrieve, and twin noisy labels in visible-infrared ReID.