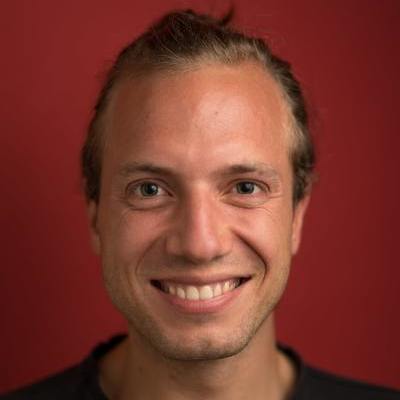
Jonas Kübler (University of Edinburgh)
Jonas Kübler is a fourth year PhD student in the department of Empirical Inference at the Max Planck Institute for Intelligent Systems. His work focuses on two-sample testing, but he also explores the use of quantum computers in machine learning.
Short Abstract: Testing whether two samples originate from the same distribution is fundamental to science and frequent in machine learning, for example, to detect distribution shifts. Tests built on the Maximum Mean Discrepancy are prominent due to their theoretical guarantees coming from kernel methods. In practice, however, choosing a suitable kernel function is crucial for good performance. The standard approach is to split the data, optimize the kernel on one part and then test on separate data. I will challenge this in two ways: First, I show that splitting the data is not necessary if, instead, one can derive a distribution that conditions on the optimization (Kübler et al. NeurIPS 2020). Second, in cases where it is necessary to split the data, it can be more convenient to directly optimize a one- dimensional witness function instead of a kernel (Kübler et al. AISTATS 2022).