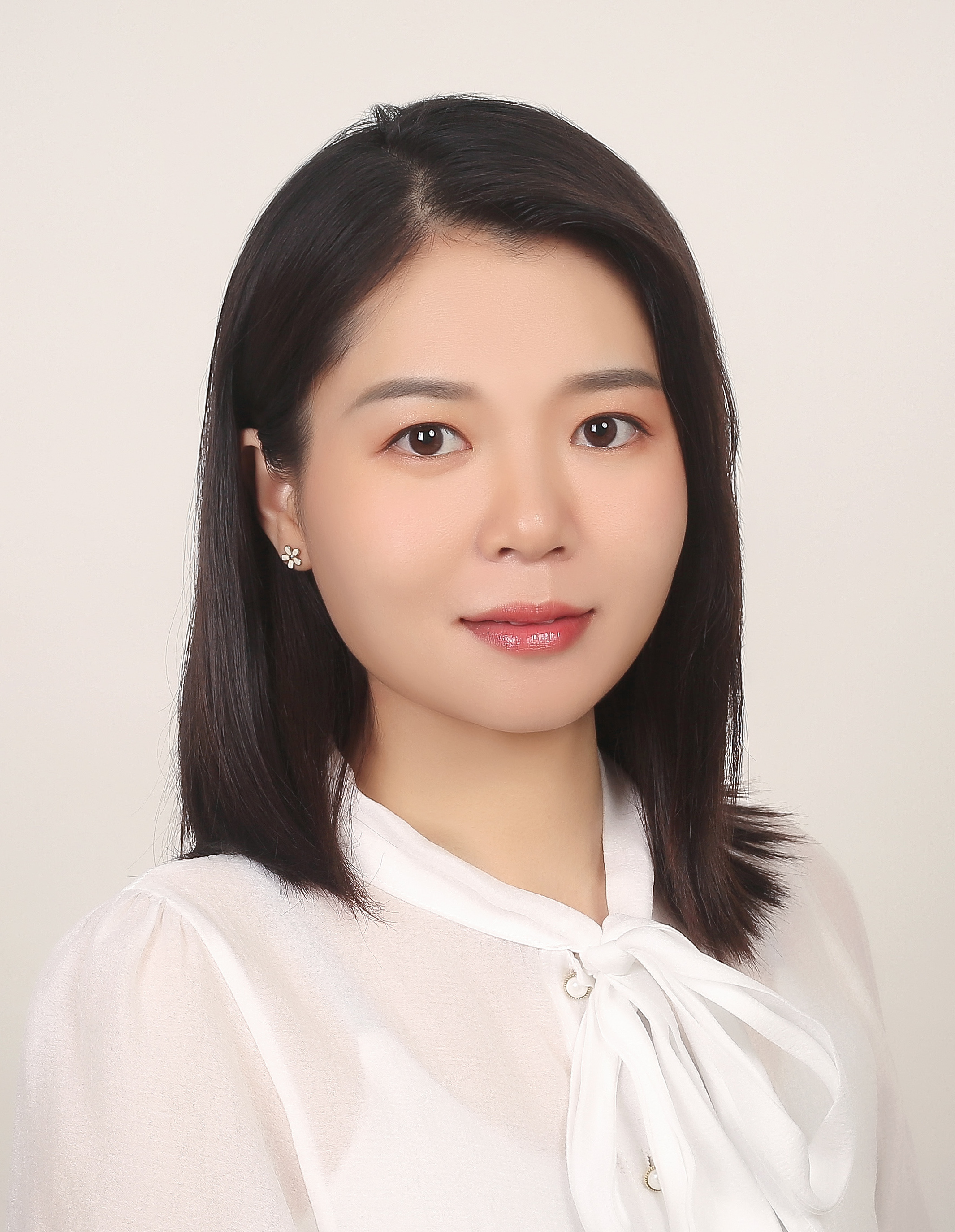
Jingjing Chen (Fudan University)
Jingjing Chen is now an associate professor at the School of Computer Science, Fudan University. Before that, she was a postdoc research fellow at the School of Computing in National University of Singapore, working with Prof. Tat-Seng Chua. She received her Ph.D. degree in Computer Science from City University of Hong Kong in July 2018, supervised by Prof. Chong-Wah Ngo. Her research interest lies in the areas of robust AI, multimedia content analysis, and deep learning. Dr. Chen won Best Student Paper Awards in ACM Multimedia 2016 and Multimedia Modeling 2017. In 2020, she was selected to the Shanghai Pujiang Talent Program.
Short Abstract: Although deep-learning based video recognition models have achieved remarkable success, they are vulnerable to adversarial examples that are generated by adding human- imperceptible perturbations on clean video samples. The existence of video adversarial examples incurs security concerns in real-world applications. Thus, it has raised increasing attention in recent years. Compared with the adversarial attack on image models, attacking video models especially under black-box setting, is more challenging. This is because the computation cost for searching the adversarial perturbations on a video is much higher due to its high dimensionality. In this talk, I will share the recent research progress of adversarial attack on video recognition models, including heuristic black-box adversarial attacks, transfer-based adversarial attacks with temporal translation as well as adversarial bullet-screen attacks.