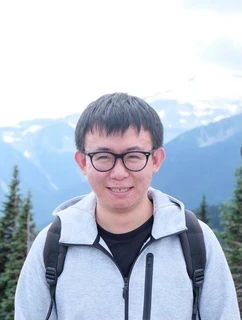
Huaxiu Yao (University of North Carolina at Chapel Hill)
Huaxiu Yao is a tenure-track Assistant Professor in the Department of Computer Science, with a joint appointment in the School of Data Science and Society at the University of North Carolina at Chapel Hill. He was previously a Postdoctoral Scholar in Computer Science at Stanford University, where he worked with Chelsea Finn. His current research focuses on developing agentic, multimodal foundation models that are widely generalizable and well-aligned with human preferences. He is also committed to applying these methods to real-world data science challenges in domains such as robotics and biomedicine. Dr. Yao has authored over 70 publications in top machine learning venues, including ICML, ICLR, and NeurIPS, and has served as a (Senior) Area Chair and workshop organizer at conferences such as ICML, NeurIPS, ICLR, ACL, and EMNLP. His research has been recognized with several honors, including the TMLR Outstanding Paper Award, the KDD 2024 Best Paper Award, the Cisco Faculty Award, AAAI 2024 New Faculty Highlights, the PharmAlliance Early Career Researcher Award, and the UNC Junior Faculty Development Award.
Short Abstract: Multimodal foundation models have transformed artificial intelligence by enabling strong generalization across a wide range of tasks and domains. However, aligning these models with specific user preferences and goals remains a major challenge. While recent advances in preference optimization have improved alignment, they often depend on large amounts of high-quality labeled data—resources that are difficult to acquire in real-world settings. In this talk, I will discuss how we can address these limitations by developing reliable reward models and generating high-quality preference data to facilitate alignment in multimodal foundation models. I will begin by highlighting why alignment is essential for improving both model performance and trustworthiness. Then, I will delve into methods for curating and synthesizing high-quality preference data, and show how post-training and self-improvement strategies can further enhance alignment. Next, I will introduce approaches for achieving stronger alignment in complex multimodal retrieval and agentic scenarios. Finally, I will show concrete examples and outline key open research directions and the challenges that remain in scaling alignment techniques across diverse multimodal applications.