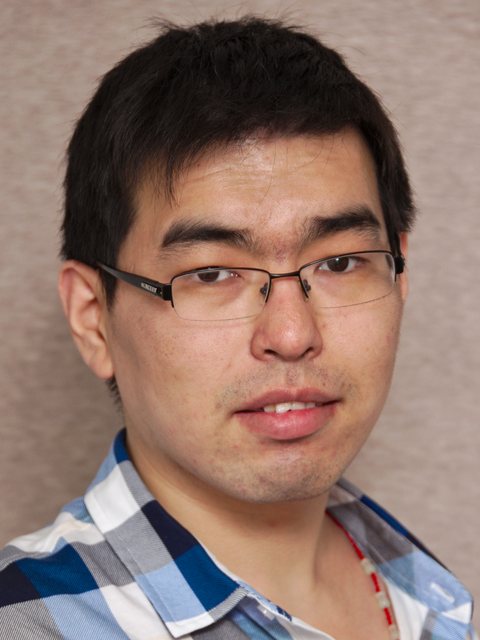
Haohan Wang (Carnegie Mellon Univerisity)
Haohan Wang is an incoming assistant professor to School of Information Science at UIUC. His research focuses on trustworthy AI and computation biology/healthcare, covering a wide spectrum of topics such as computer vision, NLP, and statistics. He recently graduated from Carnegie Mellon University, where he works with Professor Eric Xing. He was recognized as the next generation of biomedicine from the Broad Institute of MIT and Harvard and a rising star from Baidu Research.
Short Abstract: Machine learning has demonstrated remarkable prediction accuracy over i.i.d data, but the accuracy often drops when tested with data from another distribution, which sparks a proliferation of studies on robust machine learning from both the empirical and the theoretical perspectives. In this talk, we will start from the statistical end to offer another view of the machine learning robustness assuming the reason behind this accuracy drop is the reliance of models on the features that are not aligned well with how a data annotator considers similar across these two datasets. We refer to these features as misaligned features. We extend the conventional generalization error bound to a new one for this setup with the knowledge of how the misaligned features are associated with the label. Our analysis offers a set of techniques for this problem, and these techniques are naturally linked to many previous methods in robust machine learning literature. With this setup, we move on the empirical end and showcase simple heuristics of combining multiple methods under the same theoretical umbrella will lead strong methods over standard benchmarks. In particular, we will discuss our recent efforts in integrating the robust machine learning methods over robustness benchmarks over the same concept of worst-case training.