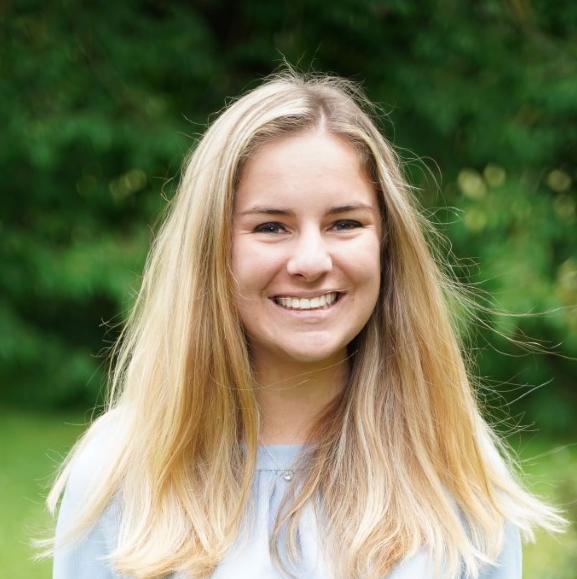
Eleonora Giunchiglia (University of Oxford)
Eleonora Giunchiglia has recently completed her PhD thesis at the University of Oxford and is currently a post-doctoral researcher at TU-Wien. Her area of research is neural-symbolic AI, with a focus on how to use neural-symbolic techniques to make deep learning models safer. Her work has been published in various top-tier conferences and journals and won her multiple awards at international venues.
Short Abstract: Multi-label classification (MC) is a standard machine learning problem in which a data point can be associated with a set of classes. A more challenging scenario is given by hierarchical multi-label classification (HMC) problems, in which every prediction must satisfy a given set of hard constraints expressing subclass relationships between classes. In this talk, we propose C-HMCNN (h), a novel approach for solving HMC problems, which, given a network h for the underlying MC problem, exploits the hierarchy information in order to produce predictions coherent with the constraints and to improve performance.