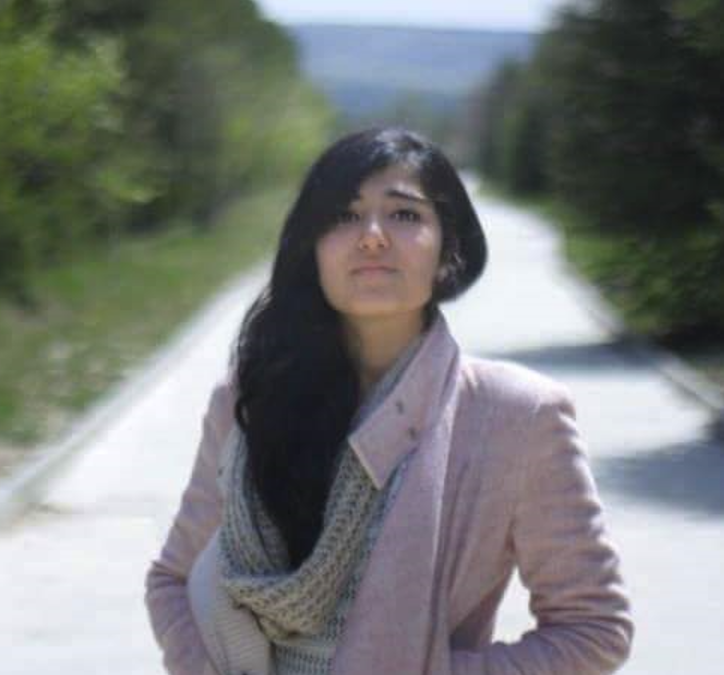
Ezgi Korkmaz (DeepMind)
Ezgi Korkmaz is a machine learning researcher and a PhD candidate in artificial intelligence and machine learning. She wrote her MSc thesis at University of California, Berkeley. Recently, she has been at DeepMind. Her research focus is robustness and generalization in machine learning.
Short Abstract:The use of deep neural networks as function approximators has led to striking progress for reinforcement learning algorithms and applications. Yet the knowledge we have on decision boundary geometry and the loss landscape of neural policies is still quite limited. In this paper, we propose a framework to investigate the decision boundary and loss landscape similarities across states and across MDPs. We conduct experiments in various games from the Arcade Learning Environment, and discover that high sensitivity directions for neural policies are correlated across MDPs. We argue that these high sensitivity directions support the hypothesis that non-robust features are shared across training environments of reinforcement learning agents. We believe our results reveal fundamental properties of the environments used in deep reinforcement learning training, and represent a tangible step towards building robust and reliable deep reinforcement learning agents.