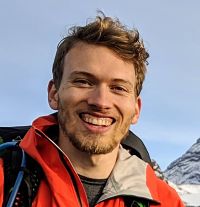
Dr. Curtis Northcutt (CEO & Co-Founder of Cleanlab)
Curtis Northcutt is CEO and cofounder of Cleanlab, an AI software company that reduces the time and cost to improve machine learning model performance. He completed his PhD at MIT, where he invented Cleanlab’s algorithms for automatically finding and fixing label issues in any dataset. He was a recipient of MIT’s Morris Levin Thesis Award, an NSF Fellowship, and a Goldwater Scholarship and has worked at several leading AI research groups including Google, Oculus, Amazon, Facebook, Microsoft, and NASA.
Short Abstract: The coupling of machine intelligence and human intelligence has the potential to empower humans with augmented capabilities (e.g., improving rhyme-density while writing song lyrics, enhancing empathy via emotion detection, and personalizing learning in online courses). Unfortunately, humans operate in an uncertain world – where the performance of even the most sophisticated model-centric artificially intelligent system often depends on its data-centric ability to deal with the uncertainty in the labels upon which it is trained.
To this end, we introduce confident learning whereby a machine (like humans) must learn with noisy-labeled data, directly quantify and identify label noise, and unlearn misconceptions by re-learning with confidence on cleaned data with erroneous labels removed. We achieve this by developing a principled theory and framework for confident learning with affordances for quantifying, identifying, and learning with label errors in data, and we open-source their implementations in the cleanlab Python package.
Based on human verification of the label errors found using cleanlab: we estimate a 3.4% lower bound error rate of the test set labels of ten of the most commonly used machine learning datasets across audio, image, and text modalities; examine the noise prevalence needed to change machine benchmark rankings; and provide corrected test sets so that humans can benchmark machine performance with increased confidence.
We'll conclude the talk with several real-world customer use cases of Cleanlab Studio, a SaaS version of the open-source package, built on top of confident learning and other related algorithmic approaches.