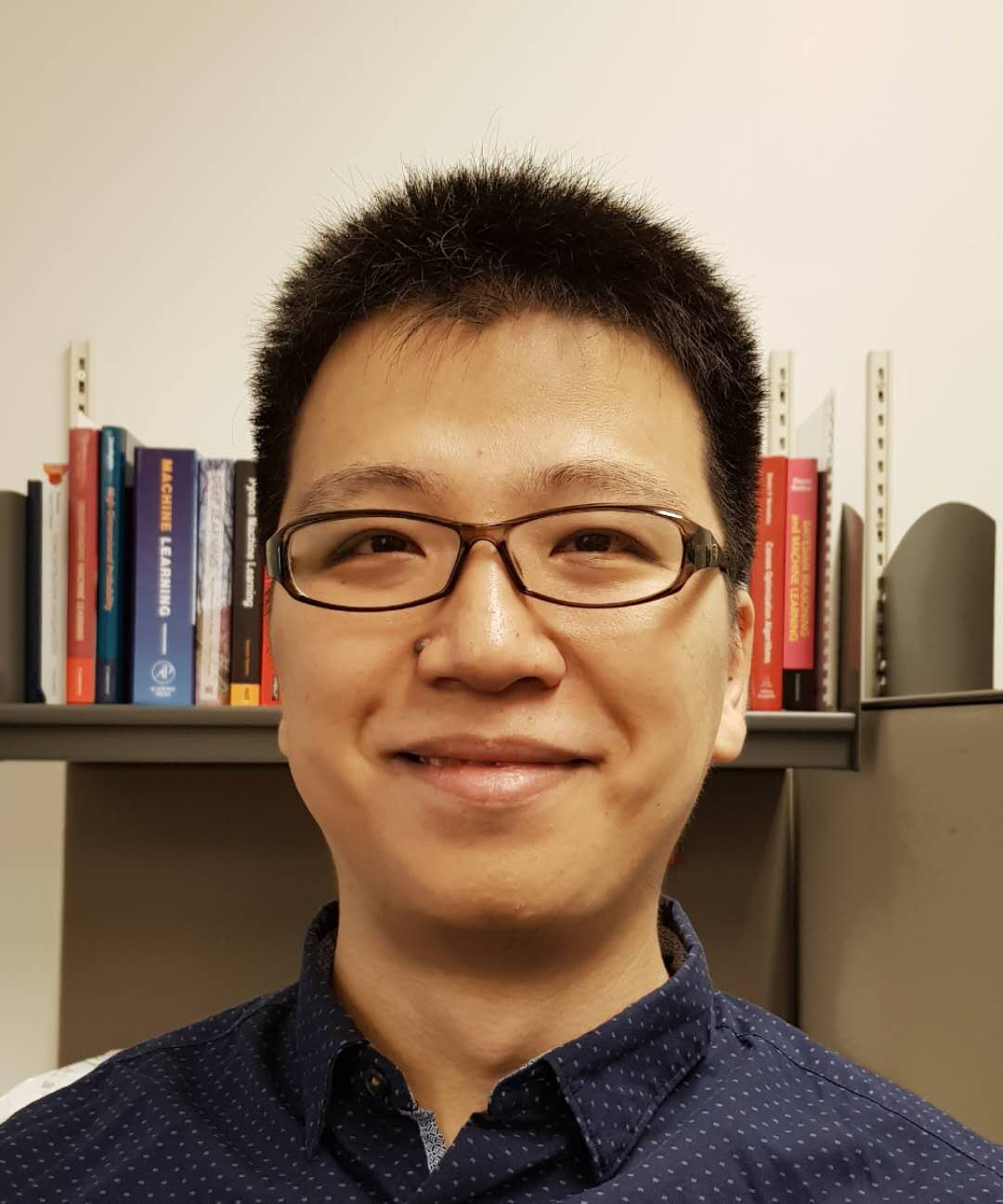
Boyu Wang (University of Western Ontario)
Boyu Wang is an Assistant Professor in the Department of Computer Science at the University of Western Ontario and an affiliated faculty member in the Vector Institute. He also holds adjunct positions in the Department of Statistical and Actuarial Sciences, the School of Biomedical Engineering, and the Brain and Mind Institute at Western. His research interests involve all aspects of machine learning: theory, algorithms, and applications. He received his Ph.D. in Computer Science from McGill University. Before joining Western in 2019, he was a postdoctoral fellow at the University of Pennsylvania and Princeton University.
Short Abstract: As machine learning techniques have achieved unprecedented success for many real-world problems it is critical to ensure that these techniques are trustworthy. In this talk, I will present three recent works on two aspects of trustworthy machine learning: robustness and fairness. Specifically, we investigate the problems of learning robust representations under distribution shift and label noise, and learning invariant representations to fulfill the sufficiency rule for fairness. By investigating both theoretical and algorithmic aspects of these problems, we have developed several principled approaches to robust and fair machine learning techniques.