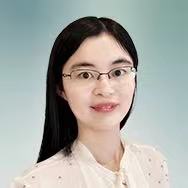
Biwei Huang (Carnegie Mellon University)
Biwei Huang is a final-year Ph.D. candidate at CMU and will be joining UCSD as an Assistant Professor in the fall of 2022. Her research interests are mainly in three aspects: (1) automated causal discovery in complex environments with theoretical guarantees, (2) advancing machine learning from the causal perspective, and (3) using or adapting causal discovery approaches to solve scientific problems. Her research contributions have been published in JMLR, ICML, NeurIPS, KDD, AAAI, IJCAI, and UAI. She successfully led a NeurIPS’20 workshop on causal discovery and causality-inspired machine learning and co-organized the first Conference on Causal Learning and Reasoning (CLeaR 2022). She is named a Rising Star of the Trustworthy ML Initiative, and is a recipient of the Presidential Fellowship at CMU in 2017 and the Apple Scholars in AI/ML PhD fellowship in 2021.
Short Abstract: Understanding causal relationships is a fundamental problem in scientific research. Recently, causal analysis has also attracted much interest in computer science and statistics. Accordingly, one focus of this talk is on causal discovery—it aims to identify causal structure and quantitative models of a large set of variables from observational (non-experimental) data, serving as a practical alternative to interventions and randomized experiments. Specifically, I will introduce recent methodological developments of causal discovery in complex environments with distribution shifts and unobserved confounders, together with successful applications. Besides learning causality, another problem of interest is how causality can help understand and advance machine learning and artificial intelligence. I will show what and how we can benefit from causal understanding to facilitate efficient, effective, and interpretable generalizations in transfer-learning tasks.