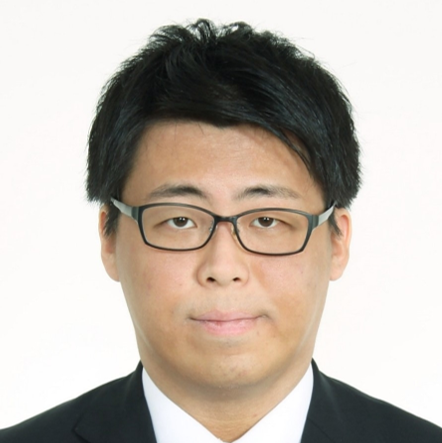
Yuki Funabiki Sony Group Corporation JAPAN
Yuki Funabiki received the B.E. and M.E. degrees from Kobe University, Japan, in 2017 and 2019. Since 2019, he has been a research scientist at the Sony Corporation, Japan. His research current interests include adversarial machine learning, image authentication and cryptography.
Short Abstract: Many researchers have focused on adversarial scenarios for machine learning, and adversarial examples are a major one among them. This talk will discuss adversarial examples against machine learning models using tabular data. This use case corresponds to fraud detection in financial transaction systems such as banking and online shopping, a scenario that is closer to real business scenarios than attacks on images. Challenges and solutions for applying the algorithm for generating adversarial examples developed in the attack on images to tabular data will be described.