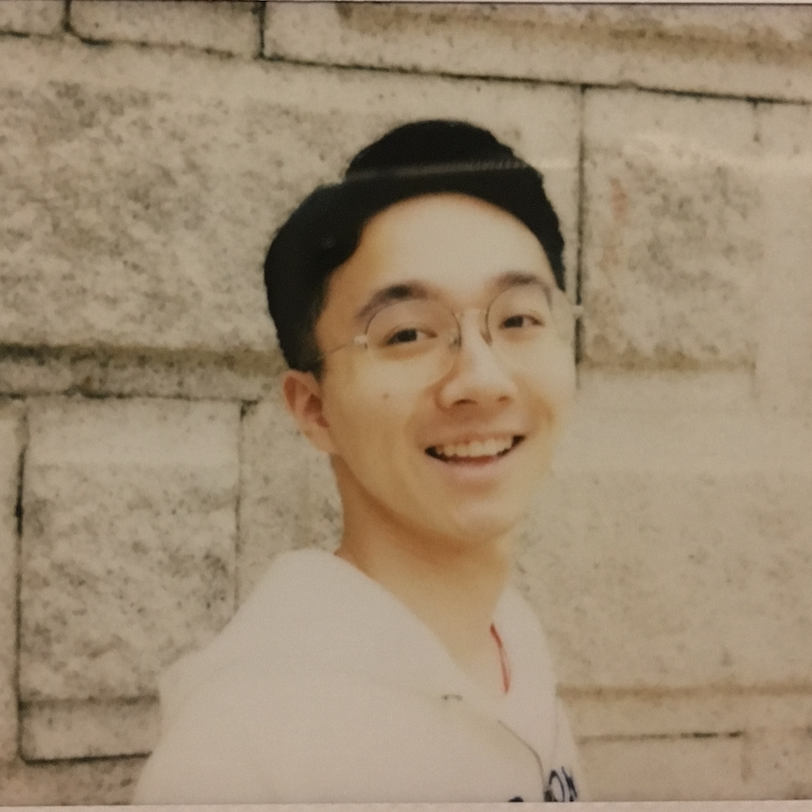
Yue Gao (University of Wisconsin at Madison)
Yue Gao is a Ph.D. candidate in the Computer Science Department at the University of Wisconsin - Madison, advised by Prof. Kassem Fawaz. His research interest broadly lies in machine learning security and system security. His current works focus on the adversarial robustness of real-world machine learning systems.
Short Abstract: Machine learning (ML) systems depend on preprocessing steps to manage diverse inputs in real-world scenarios. However, the vulnerabilities of standard and even defensive preprocessing steps are often overlooked in adversarial ML research. In this talk, we will first discuss the interplay between the vulnerabilities of standard image scaling algorithms and downstream models in a black-box setting, emphasizing how this interaction compromises robust defenses designed for individual components. After that, we will explore the limitations of preprocessing defenses aimed at providing white-box adversarial robustness. Despite increasing efforts to enhance defenses through more complex transformations, these defenses may be fundamentally flawed, necessitating a renewed understanding of their effectiveness. By addressing these vulnerabilities, we aim to offer guidance and insights for future research on preprocessing steps in real-world ML systems.