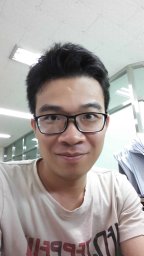
Tuan Dam (TU Darmstadt)
Tuan is a fourth year Ph.D. researcher for the Intelligent Autonomous Systems Group at TU Darmstadt. Tuan has an interdisciplinary background in Computer Science from a bachelor's in Vietnam. Tuan did his Master's thesis in Electronics and Computer Engineering at Hanyang University, Korea. He gains lots of research experience mainly in Computer Vision, Interpreting and Understanding Deep Convolution Neural Network, Entropy Regularization Markov Decision Process, and Embedded System in Academy (ESOS lab - Korea, HMI lab - Vietnam, DFKI - Berlin, Germany, Auburn University, USA) and Industry. During his Ph.D., Tuan is researching the development of principled methods that allow robots to operate in unstructured partially observable real-world environments. In his recent work, He proposed a framework to apply Partially Observable Markov Decision Process (POMDP) in Monte Carlo Planning settings. His work has been accepted to publish at the IJCAI 2020 conference with an acceptance rate of 12,6%, and ICML 2021. He is now focusing on bringing his framework to apply to robot planning problems such as Disentangling and Mikado Tasks.
Short Abstract: Monte-Carlo Tree Search (MCTS) is a class of methods for solving complex decision-making problems through the synergy of Monte-Carlo planning and Reinforcement Learning (RL). The highly combinatorial nature of the problems commonly addressed by MCTS requires the use of efficient exploration strategies for navigating the planning tree and quickly convergent value backup methods. These crucial problems are particularly evident in recent advances that combine MCTS with deep neural networks for function approximation. In this work, we propose two methods for improving the convergence rate and exploration based on a newly introduced backup operator and entropy regularization. We provide strong theoretical guarantees to bound convergence rate, approximation error, and regret of our methods. Moreover, we introduce a mathematical framework based on the use of the $\alpha$-divergence for backup and exploration in MCTS. We show that this theoretical formulation unifies different approaches, including our newly introduced ones, under the same mathematical framework, allowing to obtain different methods by simply changing the value of $\alpha$. In practice, our unified perspective offers a flexible way to balance between exploration and exploitation by tuning the single $\alpha$ parameter according to the problem at hand. We validate our methods through a rigorous empirical study from basic toy problems to the complex Atari games, and including both MDP and POMDP problems