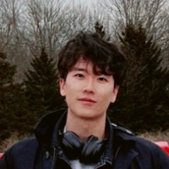
Sheng Liu (New York University)
I am a Ph.D. student in the Center for Data Science at New York University. My research interests lie in general area of machine learning, particularly in robust deep learning with imperfect datasets (corrupted data, limited supervision, small data, etc.) as well as their applications in medicine such as Alzheimer’s automatic detection. I am also a member of the Math and Data (MAD) group at NYU where I work on inverse problems and optimization. Out of school, I love playing tennis, scuba diving, surfing and any water sports.
Short Abstract:Recently, over-parameterized deep networks or large models, with increasingly more network parameters than training samples, have dominated the performances in modern machine learning. However, it has been well-known that over-parameterized networks tend to overfit and not generalize when trained on finite data. In probability estimation, the network is trained on observed outcomes of an event to estimate the probabilities of that event, leading to the network memorizing the observed outcomes completely and the estimated probabilities collapse to 0 or 1. Similarly, when learning with noisy labels, the network memorizes the wrong labels resulting in non-optimal decision rules. Yet before overfitting, the networks can learn useful information, known as early learning. Estimating probabilities reliably and being robust to noisy labels during training is of crucial importance in providing trustworthy predictions in many real-world applications with inherent uncertainty and poor label quality. In this talk, we will discuss the early learning phenomenon in probability estimation and noisy label learning, and how it can be utilized to prevent overfitting.