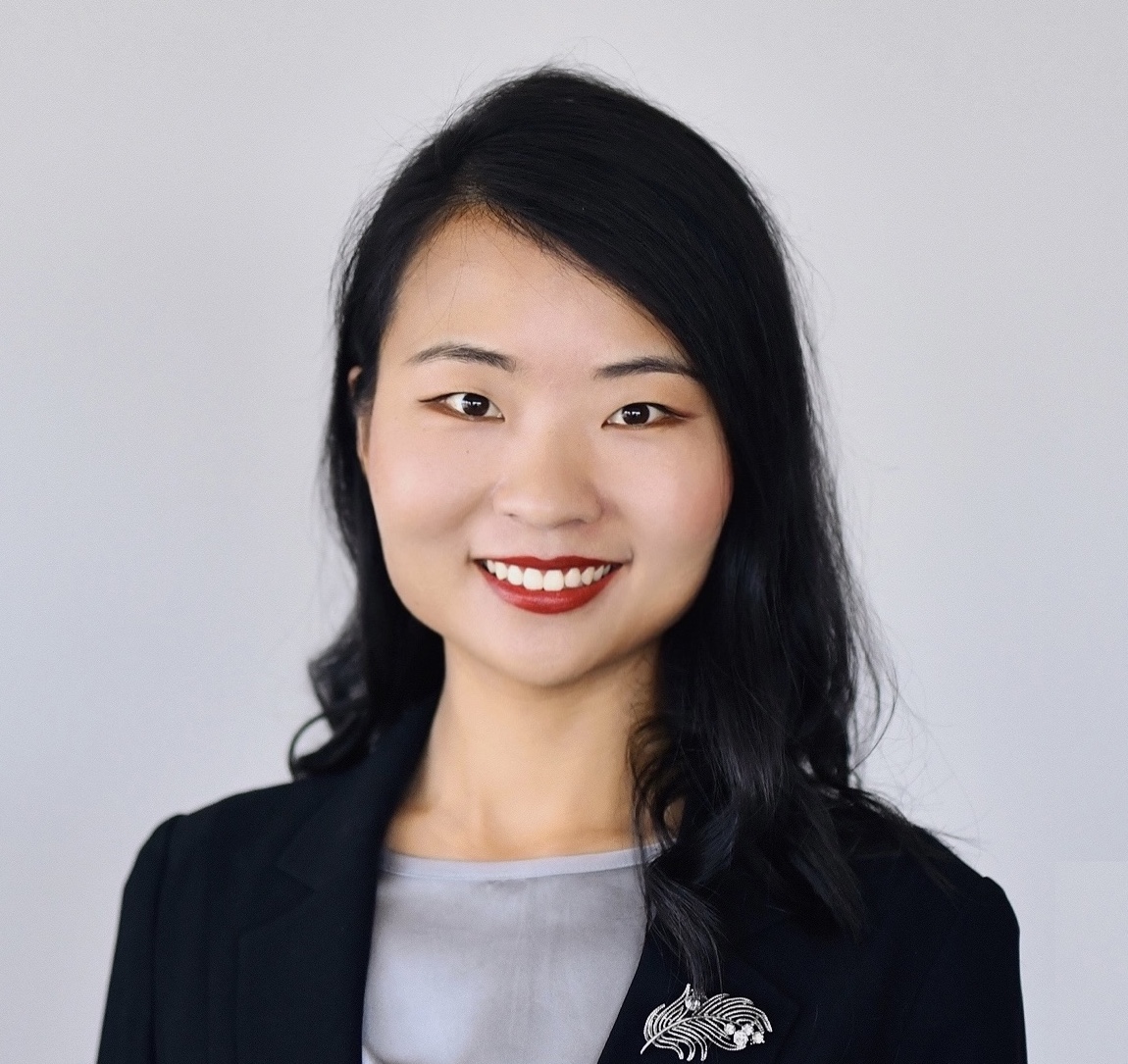
Sharon Yixuan Li (University of Wisconsin Madison)
Sharon Yixuan Li is an Assistant Professor in the Department of Computer Sciences at the University of Wisconsin Madison. Previously she was as a postdoc research fellow in the Computer Science department at Stanford AI Lab. She completed her Ph.D. from Cornell University, where she was advised by John E. Hopcroft. She has led the organization of the ICML workshop on Uncertainty and Robustness in Deep Learning in 2019 and 2020, and has served as area chair for NeurIPS, ICML, ICLR and AAAI. Her broad research interests are in deep learning and machine learning. Her research focuses on developing machine learning algorithms that can function safely and reliably in the open world. She is the recipient of Facebook Research Award, Google-Initated Research Award, Amazon Research Award, and was named Forbes 30Under30 in Science.
Short Abstract:The real world is open and full of unknowns, presenting significant challenges for machine learning (ML) systems that must reliably handle diverse, and sometimes anomalous inputs. Out-of-distribution (OOD) uncertainty arises when a machine learning model sees a test-time input that differs from its training data, and thus should not be predicted by the model. As ML is used for more safety-critical domains, the abilities to handle out-of-distribution data are central in building open-world learning systems. In this talk, I will talk about challenges, research progress and and future opportunities in detecting OOD samples for safe and reliable predictions in an open world.