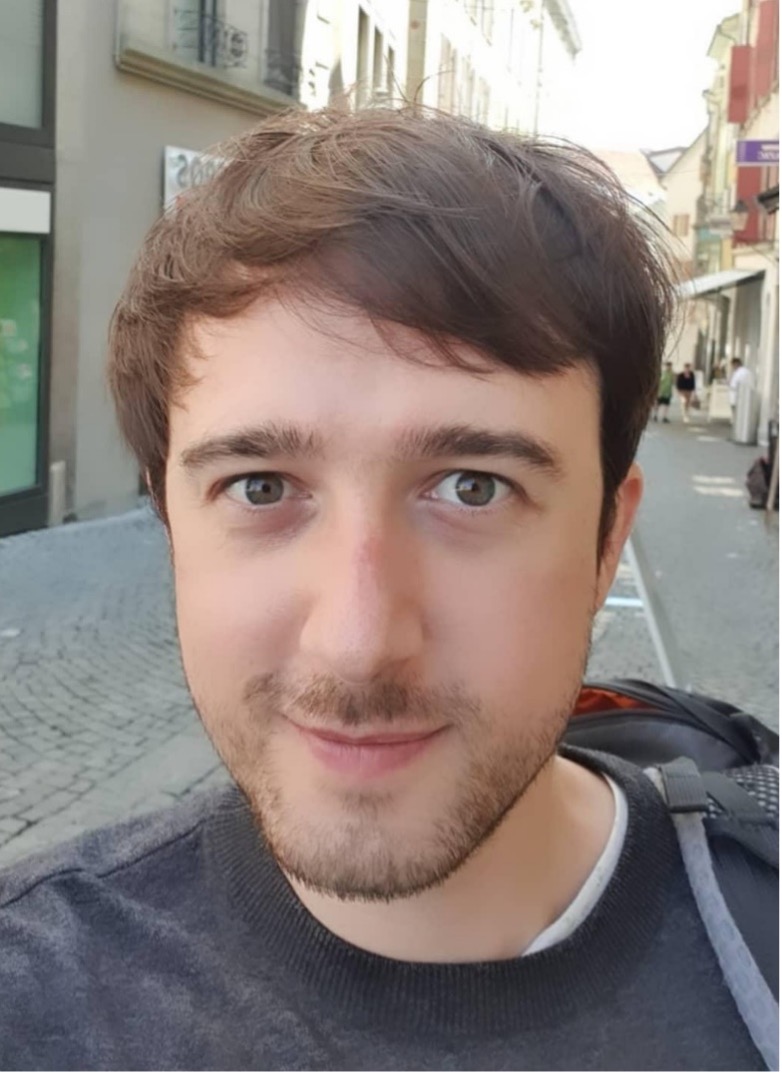
Robert Baldock (Aleph Alpha)
Robert Baldock is a Senior Researcher at Aleph Alpha in Heidelberg, Germany, where he works on language (and multi-modal) modelling. He has previously worked on the science of deep learning at Google Brain in Zurich, Switzerland, and on Bayesian Computational approaches in statistical physics as a research fellow at EPFL in Lausanne, Switzerland and a postdoc at the University of Cambridge, UK. He trained as a theoretical physicist at the University of Cambridge.
Short Abstract: Existing work on understanding deep learning often employs measures that compress all data-dependent information into a few numbers. In this work, we adopt a perspective based on the role of individual examples. We introduce a measure of the computational difficulty of making a prediction for a given input: the (effective) prediction depth. Our extensive investigation reveals surprising yet simple relationships between the prediction depth of a given input and the model’s uncertainty, confidence, accuracy and speed of learning for that data point. We further categorize difficult examples into three interpretable groups, demonstrate how these groups are processed differently inside deep models and showcase how this understanding allows us to improve prediction accuracy. Insights from our study lead to a coherent view of a number of separately reported phenomena in the literature: early layers generalize while later layers memorize; early layers converge faster and networks learn easy data and simple functions first.