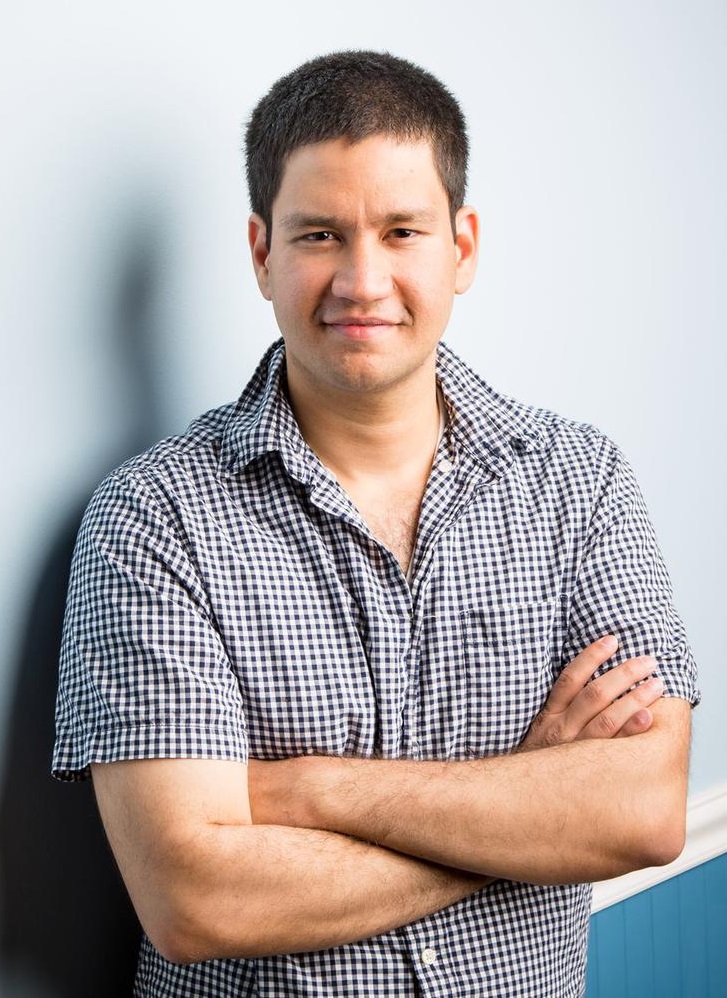
Praneeth Vepakomma (MIT)
Praneeth Vepakomma is currently a PhD student at MIT. His main research page is @ https://praneeth.mit.edu. His research focuses on developing algorithms for distributed computation in statistics & machine learning under constraints of privacy, communication & efficiency. He won the Meta PhD research fellowship, was selected as a SERC Scholar (Social and Ethical Responsibilities of Computing Scholar) by MIT’s Schwarzman College of Computing, a Baidu Best Paper Award at NeurIPS 2020-SpicyFL and a Mukh Best Paper Runner-Up Award at IEEE FG-2021. He was previously a scientist at Apple (intern), Amazon/AWS, Motorola Solutions, PublicEngines, Corning (intern) and various startups, all of which were eventually acquired.
Short Abstract: We introduce a differentially private method to measure nonlinear correlations between sensitive data hosted across two entities. We provide utility guarantees of our private estimator. Ours is the first such private estimator of nonlinear correlations within a multi-party setup. The important measure of nonlinear correlation that we privatize is that of distance correlation. Several methods use distance correlation, or a post-processing of distance correlation for feature screening, independence testing, k-sample testing, causal direction estimation, copula based data synthesis in addition to exploratory data analysis. Thereby a private measurement of distance correlation between data variables hosted on two parties, is key in order to perform these applications in a privatized manner in distributed setups. With correlations being fundamental to statistics and data sciences, this work thereby has a multitude of downstream applications beyond the above-mentioned examples.