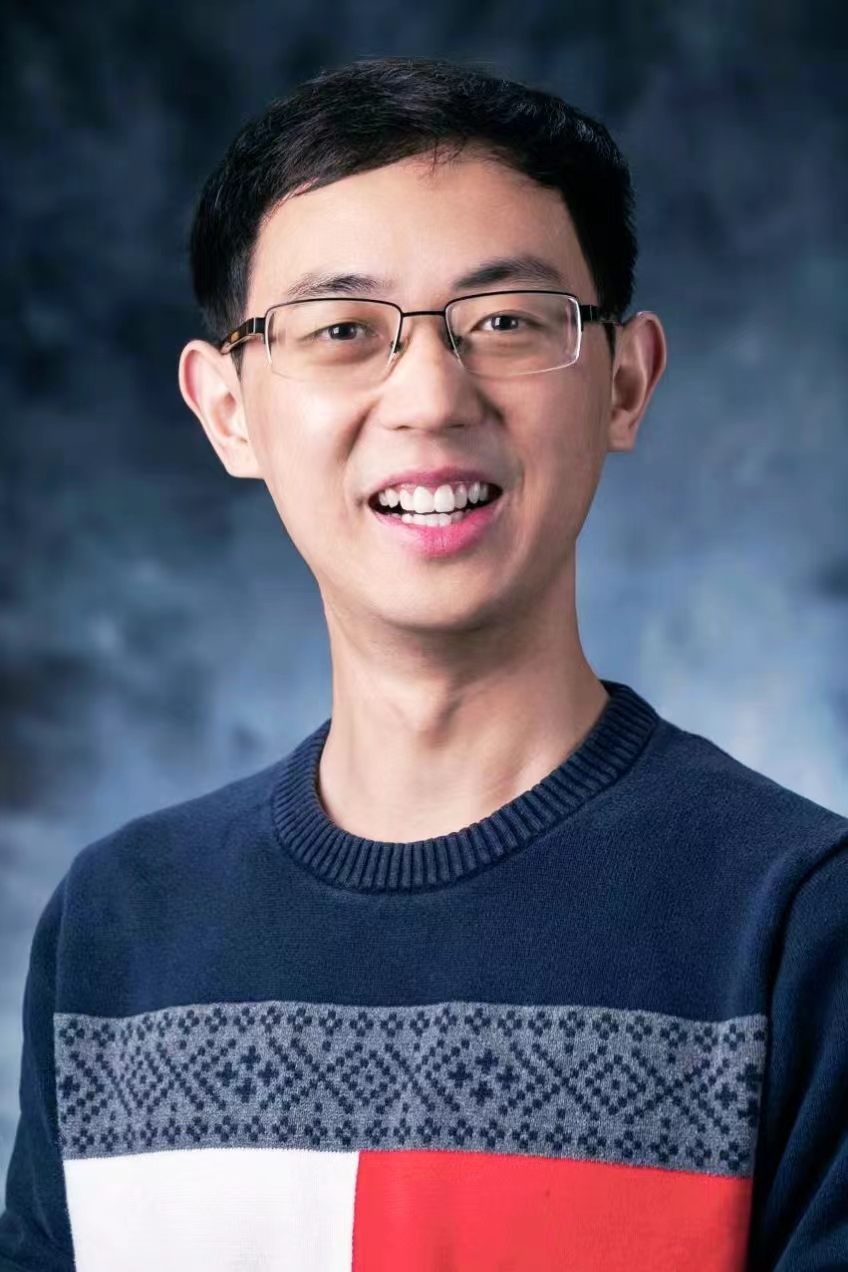
Ming Yin (UC Santa Barbara)
Ming Yin recently received his Ph.D. from the Department of Statistics and Applied Probability at UCSB, and he is currently a computer science Ph.D. candidate at UCSB. His research spans a wide range of machine learning topics, including reinforcement learning, robust ML, and Bayesian statistics. Ming serves on the program committees of several conferences including NeurIPS, ICML, AISTATS, UAI, and AAAI, and he is an Area Chair for NeurIPS 2023. He has also spent time at Princeton University and Amazon AWS AI.
Short Abstract: Reinforcement Learning has become the go-to solution for solving many sequential decision-making problems. Specifically, for many high stake real-world problems, exploration is prohibited and offline reinforcement learning is the central framework for real-life applications where online interaction is not feasible. In such cases, data is often scarce, and sample complexity is a major concern. In this talk, I will present the primary challenges of offline RL and highlight my recent efforts to address these issues. I will reveal how various techniques can enhance sample efficiency and how they can adapt to the complexity of individual problems. Additionally, we will examine the relationship between these methodologies and practical applications and outline potential avenues for future work. References: https://arxiv.org/pdf/2110.08695.pdf,https://arxiv.org/pdf/2203.05804.pdf.