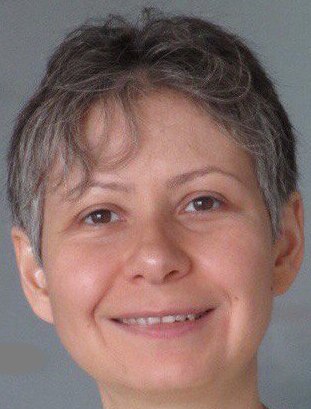
Mahsa Baktashmotlagh (University of Queensland)
Dr. Mahsa Baktash is currently employedas a senior lecturer in the School of Information Technology and Electrical Engineering (ITEE) at The University of Queensland (UQ). Her expertise and contributions in the machine learning field, especially in the areas of domain adaptation and stationarity analysis have been internationally recognized as evidenced by her track record of high-impact publications since the start of her PhD study. She has published around 50 articles, predominantly as a first author and a lead author, in major computer vision and machine learning conferences and journals mostly, including papers in top-tier computer vision (CVPR, ICCV, ECCV, BMCV, WACV) and machine learning (ICLR, ICML, ACL, AAAI, IJCAI, COLING, SDM) conferences and journals (TPAMI, JMLR, PR, IEEE Transactions on Computational Imaging).
Short Abstract: Over the years, a large number of techniques have been proposed to overcome the domain shift, which is a problem of training and test data following different distributions. These techniques can be roughly grouped into two categories: Those that perform domain adaptation and those that tackle domain generalization. The former work under the assumption of observing two domains, source and target (i.e. training and test) and having access to data from both these domains during training, albeit unannotated data in the target case. By contrast, the latter make use of labeled data from multiple source domains during training and aim to generalize to unseen target data from yet another domain at test time. I will talk about our recent works in the area of domain adaptation and domain generalization.