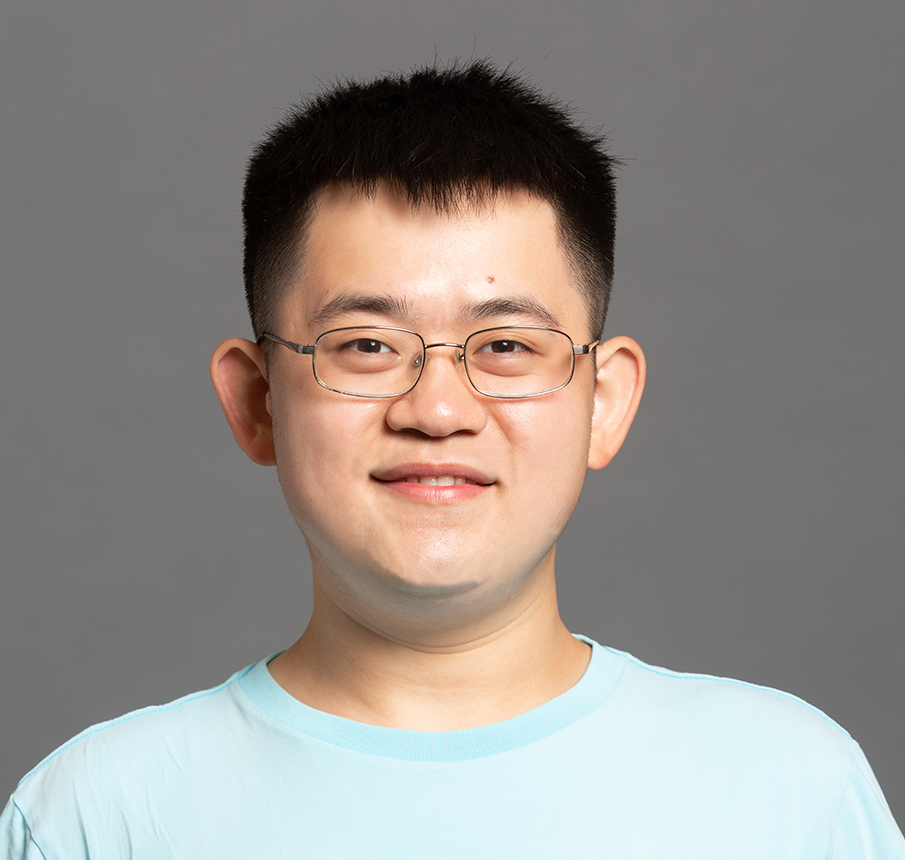
Linyi Li (UIUC)
Linyi Li is a fifth-year PhD student in computer science at University of Illinois Urbana-Champaign. He is co-advised by Bo Li and Tao Xie. His research lies in the intersection of deep learning, security, and software engineering. Specifically, he designs algorithms for certifying desired properties (such as robustness) of deep neural networks and methods for training certifiable deep neural networks. He is a finalist of Two Sigma PhD Fellowship and Qualcomm Innovation Fellowship, and a recipient of Wing Kai Cheng Fellowship at UIUC. Prior to UIUC, he received his bachelor's degree in computer science from Tsinghua University, China.
Short Abstract: Given the rising security concerns for modern deep learning systems in deployment, designing certifiable large-scale deep learning systems for real-world requirements is in urgent demand. This talk introduces our series of work on constructing certifiable large-scale deep learning systems towards trustworthy machine learning, achieving robustness against Lp perturbations, semantic transformations, poisoning attacks, distributional shifts; fairness; and reliability against numerical defects. Then, I will share core methodologies for designing certifiable deep learning systems including diversity-enabled training, efficient model abstraction, threat-model-dependent smoothing, and precise worse-case characterization. At the end of the talk, I will summarize several challenges that impede the large-scale deployment of certifiable deep learning and discuss future directions.