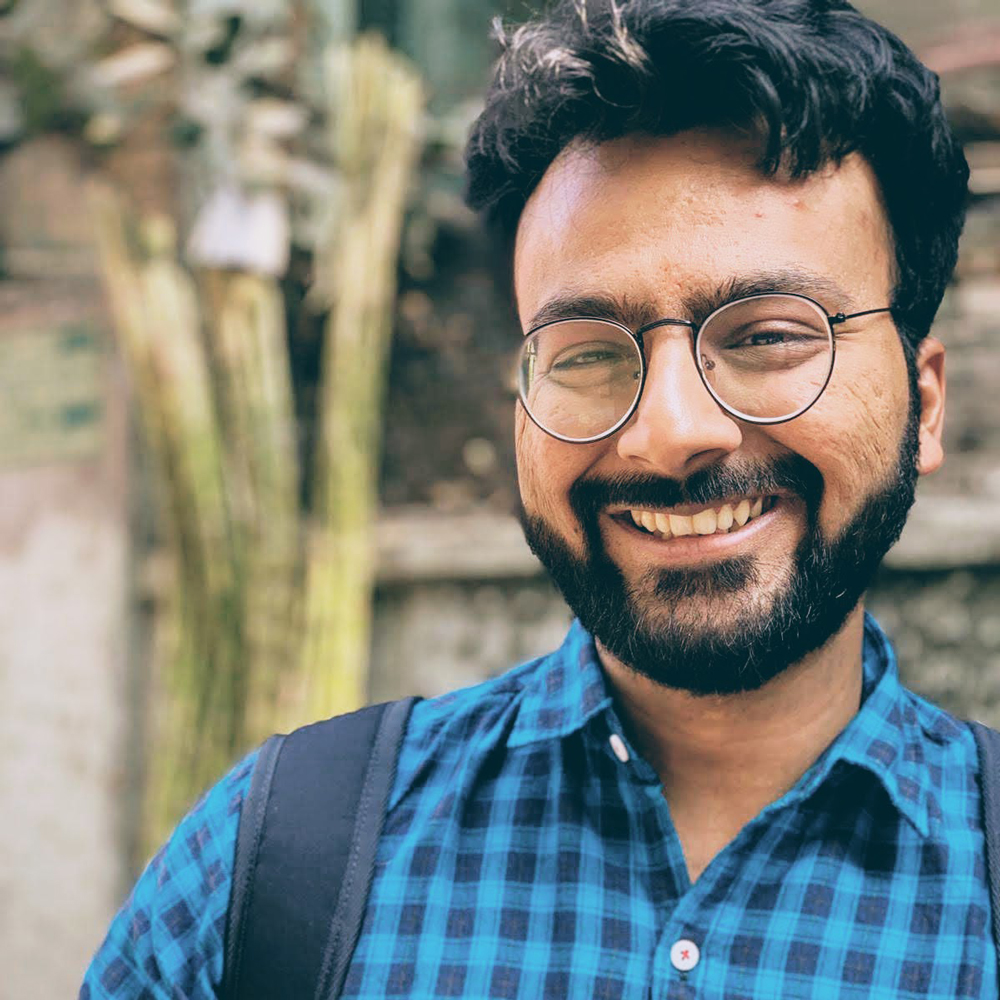
Chirag Agarwal (Adobe Media, Data Science Research Lab)
Chirag is a Research Scientist at Adobe Media and Data Science Research Lab and a research affiliate at Harvard University. His research interest includes developing trustworthy machine learning that goes beyond training models for specific downstream tasks and ensuring they satisfy other desirable properties, such as explainability, fairness, and robustness. He is one of the co-founders of the Trustworthy ML Initiative, a forum and seminar series related to Trustworthy ML, and an active member of the Machine Learning Collective research group that focuses on democratizing research by supporting open collaboration in machine learning (ML) research. His works have been published in top machine learning, artificial intelligence, and computer vision conferences, including ICML, AISTATS, UAI, and CVPR.
Short Abstract: In machine learning, a question of great interest is understanding what examples are challenging for a model to classify and what are the advantages of identifying such challenging examples. Identifying atypical examples ensures the safe deployment of models, isolates samples that require further human inspection, and provides interpretability into model behavior. In this talk, we will be discussing i) Variance of Gradients (VoG), a valuable and efficient metric to rank data by difficulty and surface a tractable subset of the most challenging examples for human-in-the-loop auditing, and ii) the utility of such harder subset in a transfer learning setting. In particular, I will be showing how harder subsets are a better measure to estimate the transferability from a source to a target dataset.