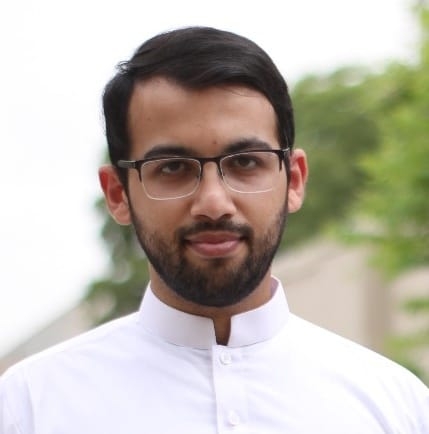
Muhammad Ahmed Shah (Carnegie Mellon University)
Muhammad Ahmed Shah is a 2nd year PhD student at Carnegie Mellon University, and is advised by Dr. Bhiksha Raj. His current research focus is developing biologically-inspired methods for making deep neural networks more robust to input corruptions, particularly adversarial attacks. In the past he has worked on a variety of research topics including machine learning privacy, neural model compression and information retrieval. His work has been published in several conferences including ICASSP, Interspeech and ICPR.
Short Abstract: Deep neural networks (DNNs) have been shown to be vulnerable to adversarial attacks -- subtle, perceptually indistinguishable perturbations of inputs that change the response of the model. In the context of vision, we hypothesize that a factor contributing to the robustness of human visual perception is our constant exposure to low-fidelity visual stimuli in our peripheral vision. To investigate this hypothesis, we develop R-Blur, an image transform that simulates the loss in fidelity of peripheral vision by blurring the image and reducing its color saturation based on the distance from a given fixation point. We show that DNNs trained on images transformed by R-Blur are substantially more robust to adversarial attacks, as well as other, non-adversarial, corruptions than DNNs trained on the original images, with the former achieving up to 69% higher accuracy on perturbed data. We further show that the robustness induced by R-Blur is certifiable.