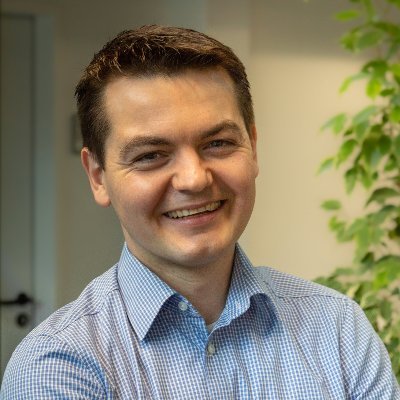
Adam Kortylewski (Max Planck Institute for Informatics)
Adam Kortylewski is a research group leader at the University of Freiburg and the Max-Planck Instutite for Informatics where he leads the Generative Computer Vision Lab. Before that he was a postdoc at Johns Hopkins University with Alan Yuille for three years. He obtained his PhD from the University of Basel with Thomas Vetter. His research focuses on studying computer vision from a generative perspective. His core working hypothesis is that vision computer vision systems need to develop a causal 3D understanding of images following an analysis-by-synthesis approach, in order to become a truly foundational component of AI systems.
Short Abstract: Deep learning sparked a tremendous increase in the performance of computer vision systems over the past decade. However, Deep Neural Networks (DNNs) are still far from reaching human-level performance at visual recognition tasks. The most important limitation of DNNs is that they fail to give reliable predictions in unseen or adverse viewing conditions, which would not fool a human observer, such as when objects are partially occluded, seen in an unusual pose or context, or in bad weather. This lack of robustness in DNNs is generally acknowledged, but the problem largely remains unsolved. In this talk, I will give an overview of the principles underlying my work on building robust deep neural networks for computer vision. My working hypothesis is that vision systems need a causal 3D understanding images by following an analysis-by-synthesis approach. I will discuss a new type of neural network architecture that implements such an approach, and I will show that these generative neural network models are vastly superior to traditional models in terms of robustness, learning efficiency and because they can solve many vision tasks at once. Finally, I will give a brief outlook on current projects of mine and future research directions.